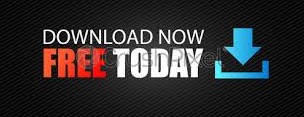

Percentage of Variance (Information) for each by PC
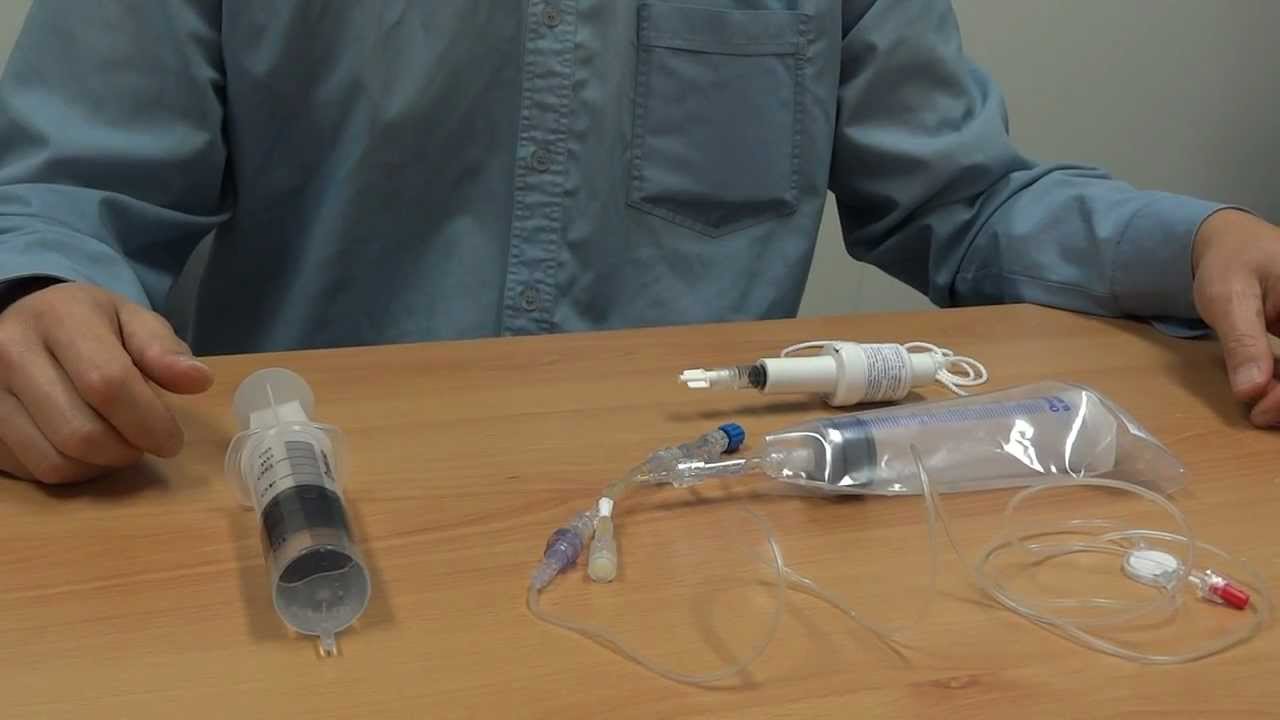
So, the idea is 10-dimensional data gives you 10 principal components, but PCA tries to put maximum possible information in the first component, then maximum remaining information in the second and so on, until having something like shown in the scree plot below. These combinations are done in such a way that the new variables (i.e., principal components) are uncorrelated and most of the information within the initial variables is squeezed or compressed into the first components. Principal components are new variables that are constructed as linear combinations or mixtures of the initial variables. Before getting to the explanation of these concepts, let’s first understand what do we mean by principal components. Step 3: Compute the eigenvectors and eigenvalues of the covariance matrix to identify the principal componentsĮigenvectors and eigenvalues are the linear algebra concepts that we need to compute from the covariance matrix in order to determine the principal components of the data. Now that we know that the covariance matrix is not more than a table that summarizes the correlations between all the possible pairs of variables, let’s move to the next step. if negative then : One increases when the other decreases (Inversely correlated).if positive then : the two variables increase or decrease together (correlated).It’s actually the sign of the covariance that matters : What do the covariances that we have as entries of the matrix tell us about the correlations between the variables? And since the covariance is commutative (Cov(a,b)=Cov(b,a)), the entries of the covariance matrix are symmetric with respect to the main diagonal, which means that the upper and the lower triangular portions are equal. Since the covariance of a variable with itself is its variance (Cov(a,a)=Var(a)), in the main diagonal (Top left to bottom right) we actually have the variances of each initial variable. For example, for a 3-dimensional data set with 3 variables x, y, and z, the covariance matrix is a 3×3 matrix of this from: Covariance Matrix for 3-Dimensional Data The covariance matrix is a p × p symmetric matrix (where p is the number of dimensions) that has as entries the covariances associated with all possible pairs of the initial variables. So, in order to identify these correlations, we compute the covariance matrix. Because sometimes, variables are highly correlated in such a way that they contain redundant information. The aim of this step is to understand how the variables of the input data set are varying from the mean with respect to each other, or in other words, to see if there is any relationship between them. Once the standardization is done, all the variables will be transformed to the same scale. Mathematically, this can be done by subtracting the mean and dividing by the standard deviation for each value of each variable. So, transforming the data to comparable scales can prevent this problem. That is, if there are large differences between the ranges of initial variables, those variables with larger ranges will dominate over those with small ranges (For example, a variable that ranges between 0 and 100 will dominate over a variable that ranges between 0 and 1), which will lead to biased results. More specifically, the reason why it is critical to perform standardization prior to PCA, is that the latter is quite sensitive regarding the variances of the initial variables. The aim of this step is to standardize the range of the continuous initial variables so that each one of them contributes equally to the analysis. Step by Step Explanation of PCA Step 1: Standardization So to sum up, the idea of PCA is simple - reduce the number of variables of a data set, while preserving as much information as possible. Because smaller data sets are easier to explore and visualize and make analyzing data much easier and faster for machine learning algorithms without extraneous variables to process. Reducing the number of variables of a data set naturally comes at the expense of accuracy, but the trick in dimensionality reduction is to trade a little accuracy for simplicity. Principal Component Analysis, or PCA, is a dimensionality-reduction method that is often used to reduce the dimensionality of large data sets, by transforming a large set of variables into a smaller one that still contains most of the information in the large set. An overview of principal component analysis (PCA) What Is Principal Component Analysis?
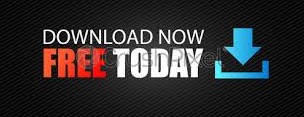